In August, Meta launched its SeamlessM4T multimodal AI translation model, which initially supported almost 100 languages for text and 36 languages for speech. Now, with an updated “v2” architecture, Meta is expanding the capabilities of SeamlessM4T to enhance conversational translations, aiming to make them more spontaneous and expressive. This is a significant advancement, addressing the challenge of achieving authentic and expressive translations, which has been a notable hurdle in cross-language conversations.
SeamlessM4T is specifically designed to provide seamless translation and transcription across various speech and text functions. It has the ability to translate almost 100 languages for speech-to-text and text-to-text functions, while also supporting speech-to-speech and text-to-speech capabilities in the same languages. Furthermore, the model can output translations in any of the other 36 languages, including English.
The first notable feature introduced in the updated SeamlessM4T is “SeamlessExpressive.” As the name suggests, this feature allows the translation of not only the words spoken but also the speaker’s expressions. These expressions encompass pitch, volume, emotional tone (such as excitement, sadness, or whispers), speech rate, and pauses. The intention is to make translated speeches sound less robotic and more natural. SeamlessExpressive is available for several languages, including English, Spanish, German, French, Italian, and Chinese.
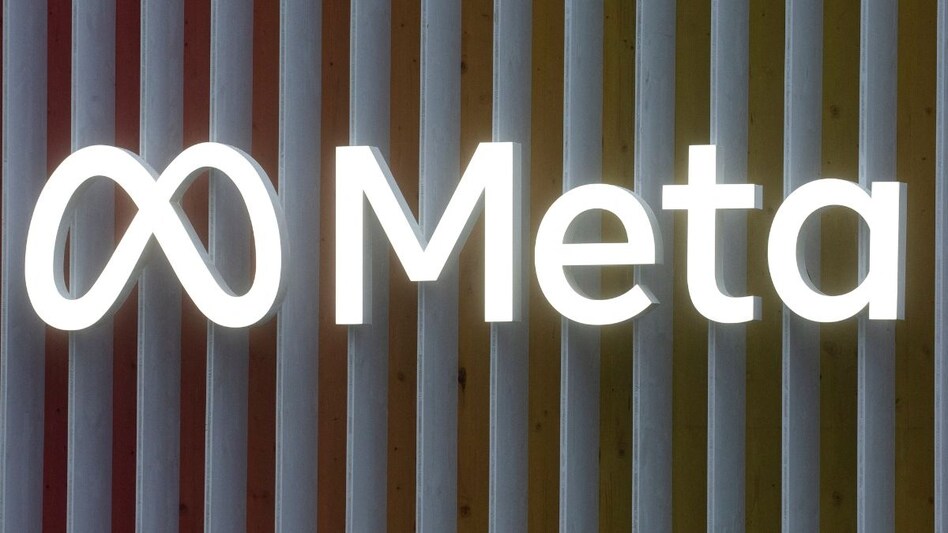
The second feature, “SeamlessStreaming,” aims to expedite the translation process during a speech. It enables the tool to begin translating a speech while the speaker is still talking, reducing the waiting time for others to hear the translation. Despite a short latency of just under two seconds, this feature eliminates the need to wait until a speaker finishes a sentence. Addressing the challenge posed by different sentence structures in various languages, Meta developed an algorithm for SeamlessStreaming to analyze partial audio input and determine whether there is sufficient context to start generating a translated output or if it should continue listening.
SeamlessM4T relies on the existing PyTorch-based multitask UnitY model architecture. This architecture already possesses the capability to perform different modal translations, including automatic speech recognition. Additionally, the model utilizes the BERT 2.0 system for audio encoding, breaking down inputs into component tokens for analysis. The HiFi-GAN unit vocoder is employed to generate spoken responses.
Overall, Meta’s updates to the SeamlessM4T model demonstrate a commitment to addressing challenges in language translation, particularly in achieving more expressive and spontaneous conversations across diverse languages. The enhanced features contribute to a more natural and authentic experience, marking a significant step forward in the field of AI-powered language translation.